本期的 15 篇论文如下:
[00:21] 🎬 Any2Caption:Interpreting Any Condition to Caption for Controllable Video Generation(Any2Caption:将任意条件解析为描述以实现可控视频生成)
[01:01] 🎬 Exploring the Effect of Reinforcement Learning on Video Understanding: Insights from SEED-Bench-R1(探索强化学习对视频理解的影响:来自SEED-Bench-R1的见解)
[01:48] ⚖ JudgeLRM: Large Reasoning Models as a Judge(JudgeLRM:将大型推理模型作为评判者)
[02:30] 🤖 CodeARC: Benchmarking Reasoning Capabilities of LLM Agents for Inductive Program Synthesis(CodeARC:用于归纳程序合成的LLM智能体推理能力基准测试)
[03:13] 💡 Open-Qwen2VL: Compute-Efficient Pre-Training of Fully-Open Multimodal LLMs on Academic Resources(Open-Qwen2VL:在学术资源上进行计算高效的完全开源多模态LLM预训练)
[04:02] 🎥 GeometryCrafter: Consistent Geometry Estimation for Open-world Videos with Diffusion Priors(GeometryCrafter:基于扩散先验的开放世界视频一致几何体估计)
[04:48] 💻 Z1: Efficient Test-time Scaling with Code(Z1:基于代码的高效测试时扩展)
[05:26] 🤖 Agent S2: A Compositional Generalist-Specialist Framework for Computer Use Agents(Agent S2:计算机使用代理的组合式通用-专家框架)
[06:08] 💃 MixerMDM: Learnable Composition of Human Motion Diffusion Models(MixerMDM:人类运动扩散模型的可学习组合)
[06:46] 🏢 Command A: An Enterprise-Ready Large Language Model(Command A:一款面向企业就绪的大型语言模型)
[07:31] 💡 Harnessing the Reasoning Economy: A Survey of Efficient Reasoning for Large Language Models(驾驭推理经济:大型语言模型高效推理的综述)
[08:09] 🎬 OmniMMI: A Comprehensive Multi-modal Interaction Benchmark in Streaming Video Contexts(OmniMMI:流视频场景下综合性多模态交互基准)
[08:53] 🤯 Recitation over Reasoning: How Cutting-Edge Language Models Can Fail on Elementary School-Level Reasoning Problems?(背诵胜于推理:顶尖语言模型如何在小学水平的推理问题上失败?)
[09:40] 🖼 Scaling Language-Free Visual Representation Learning(扩展无语言视觉表征学习)
[10:23] 🤔 When To Solve, When To Verify: Compute-Optimal Problem Solving and Generative Verification for LLM Reasoning(何时求解,何时验证:LLM推理的计算最优问题求解与生成式验证)

【关注我们】
您还可以在以下平台找到我们,获得播客内容以外更多信息
小红书: AI速递
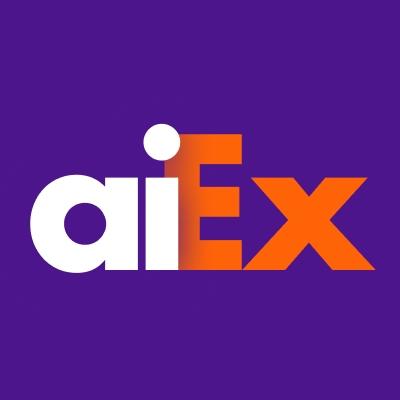
空空如也
暂无小宇宙热门评论