本期的 21 篇论文如下:
[00:26] 🚀 Breaking the Memory Barrier: Near Infinite Batch Size Scaling for Contrastive Loss(打破内存壁垒:对比损失的近无限批量规模扩展)
[01:09] 🔄 LOGO -- Long cOntext aliGnment via efficient preference Optimization(LOGO -- 通过高效偏好优化实现长上下文对齐)
[01:45] 🧠 Unleashing Reasoning Capability of LLMs via Scalable Question Synthesis from Scratch(从零开始释放LLMs的推理能力:可扩展的问题合成方法)
[02:30] 🤔 Can Knowledge Editing Really Correct Hallucinations?(知识编辑真的能纠正幻觉吗?)
[03:17] 🎮 Unbounded: A Generative Infinite Game of Character Life Simulation(无界:生成式无限角色生活模拟游戏)
[04:02] 🎥 Framer: Interactive Frame Interpolation(Framer:交互式帧插值)
[04:48] 📊 Distill Visual Chart Reasoning Ability from LLMs to MLLMs(从LLMs到MLLMs的视觉图表推理能力提炼)
[05:35] 📉 Why Does the Effective Context Length of LLMs Fall Short?(为什么大型语言模型的有效上下文长度不足?)
[06:14] 🔒 Robust Watermarking Using Generative Priors Against Image Editing: From Benchmarking to Advances(基于生成先验的鲁棒水印技术对抗图像编辑:从基准测试到进展)
[06:52] 🔧 Skywork-Reward: Bag of Tricks for Reward Modeling in LLMs(天工奖励:LLM奖励建模的技巧包)
[07:27] 🌍 CAMEL-Bench: A Comprehensive Arabic LMM Benchmark(CAMEL-Bench:一个全面的阿拉伯语大型多模态模型基准)
[08:09] 📊 Should We Really Edit Language Models? On the Evaluation of Edited Language Models(我们真的应该编辑语言模型吗?关于编辑语言模型的评估)
[08:43] 🌐 ADEM-VL: Adaptive and Embedded Fusion for Efficient Vision-Language Tuning(ADEM-VL:高效视觉语言调优的自适应嵌入融合方法)
[09:20] 🌐 WAFFLE: Multi-Modal Model for Automated Front-End Development(WAFFLE:自动化前端开发的多模态模型)
[09:52] 📚 CCI3.0-HQ: a large-scale Chinese dataset of high quality designed for pre-training large language models(CCI3.0-HQ:一个用于预训练大型语言模型的高质量大规模中文数据集)
[10:30] 🔄 Stable Consistency Tuning: Understanding and Improving Consistency Models(稳定一致性调优:理解与改进一致性模型)
[11:10] 🧮 Language Models are Symbolic Learners in Arithmetic(语言模型在算术中的符号学习者角色)
[12:00] 🐍 Taipan: Efficient and Expressive State Space Language Models with Selective Attention(Taipan:高效且表达丰富的状态空间语言模型与选择性注意力)
[12:44] 🔄 Value Residual Learning For Alleviating Attention Concentration In Transformers(残差值学习缓解Transformer中的注意力集中问题)
[13:23] 📚 Multi-Draft Speculative Sampling: Canonical Architectures and Theoretical Limits(多草稿推测采样:典型架构与理论极限)
[14:03] 🤖 Data Scaling Laws in Imitation Learning for Robotic Manipulation(机器人操作中的模仿学习数据缩放定律)

【关注我们】
您还可以在以下平台找到我们,获得播客内容以外更多信息
小红书: AI速递
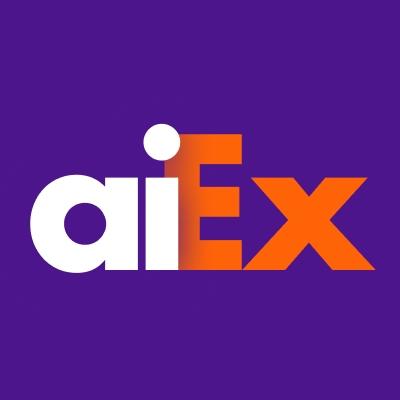
空空如也
暂无小宇宙热门评论